Technology
Applying Artificial Intelligence in Wealth Management: Compelling Use Cases Across The Client Life Cycle
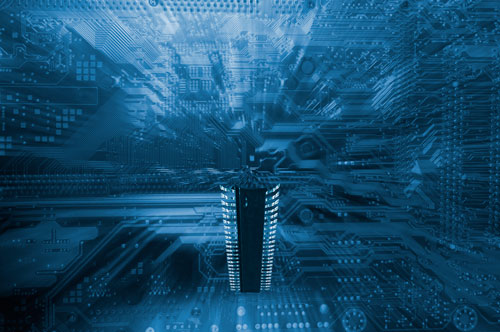
The publisher of this news service, alongside EY and Finantix, is producing a major report on what AI means for wealth manager, going deep into practical details to provide real, actionable ideas for the sector. A number of segments from the report are being run on this service.
The publisher of this news service is, in conjunction
with technology firm Finantix and professional services, audit
and accountancy group EY, issuing a research report exploring how
artificial intelligence is likely to affect wealth management
continue to do so. This publication will release chapters from
the report in coming days and weeks. We hope these articles
encourage readers to obtain the whole copy to view its mass of
data and charts alongside text. The material has been produced by
the ClearView Financial Media team, led by Wendy Spires, head of
research at ClearView. Wendy writes the first chapter. Before
getting to her article, here are key terms and concepts around
artificial intelligence that readers will find useful. (To
download the full report and background, click
here.)
Natural Language Processing
Natural Language Processing (NLP) is a component of AI whereby
computer programmes are able to understand human speech as it is
spoken.
Computers have traditionally required humans to communicate with
them in a programming language that is precise, unambiguous and
highly structured. The first task of NLP is to understand
the natural language received by the computer and then convert
this into a programming language, breaking it down into tiny
units. This process identifies words in their grammatical forms
as nouns, verbs, adjectives, past tense, etc. In the last stage,
the computer programming language is converted into an audible or
textual format for the user.
The ultimate goal of NLP is to do away with computer programming
languages altogether. Instead of specialised languages such as
Java, Ruby or C, there would only be "human" language, with the
software built to analyse, understand and generate human
languages naturally.
Applications of NLP
Machine Translation: The challenge of making the world's
information accessible to everyone has simply outgrown the
capacity for human translation. The challenge with machine
translation technologies is not in translating words, but in
preserving the meaning of sentences. Hermeneutics is a complex
technological (and arguably philosophical) issue that is at the
heart of NLP.
Fighting Spam: The false-positive and false-negative issues of
spam filters are at the heart of NLP technology, again boiling
down to the challenge of extracting meaning from strings of
text.
Question Answering: A big focus of efforts in NLP has been in
enabling tools like chatbots to recognise natural language
questions, extract their meaning and provide the right answer
automatically.
Automated reasoning
Automated reasoning is an area of computer science and
mathematical logic, producing computer programmes that reason
automatically. The first step in performing automated
reasoning is to express a statement of theorems in a formal
language. The second step is to use automated algorithmic
manipulations on those formal expressions.
Software development is one of the key reasons for using
Automated Reasoning. We can’t afford errors in software
development as this will affect far-reaching applications of
computer programming such as air traffic control, banking and
utilities. Errors are annoying, expensive and can even result in
the loss of lives.
Applications of Automated Reasoning:
Research: Automated reasoning checks the correctness of proofs in
mathematics, supplementing or even replacing the existing ‘social
process’ of peer review etc. with a more objective
criterion.
Testing Computers: Can be used to extend rigorous proof from pure
mathematics to the verification of computer systems (programs,
hardware systems, protocols etc.).
Machine Learning/Analytics
Machine Learning is a type of AI that predicts outcomes without
explicit programming. Supervised algorithms require humans to
provide both input and desired output, in addition to furnishing
feedback about the accuracy of predictions during training. Once
training is complete, the algorithm will apply what was learned
to new data. Unsupervised algorithms do not need to be trained
with desired outcome data. Instead, they use an iterative
approach called deep learning to review data and arrive at
conclusions.
For example, a computer might be provided with a teaching set of photographs, some of which say, “this is a cat” and some of which say, “this is not a cat”. Then, shown a series of new photos, it would begin to identify which photos were of cats independently, getting “smarter” at completing its task over time.
Applications of Machine Learning:
Data Security: Malware is a huge problem. But each piece of new
malware tends to have almost the same code as previous versions —
only between 2 and 10 per cent of the files change from
iteration to iteration. Machine Learning algorithms can predict
which files are malware with great accuracy.
Financial Trading: Many prestigious trading
firms use proprietary systems to predict and execute trades at
high speeds and high volume. Many of these rely on probabilities,
but even a trade with a relatively low probability, at a high
enough volume or speed, can turn huge profits for the
firms.
Chapter 1: The Real Role of AI and Why Wealth Managers
Have Less to Fear Than They Might Think
Statistics about the financial services industry’s accelerating
reliance on technology may well strike fear into the hearts of
its professionals. For instance, it has been estimated that 30
per cent of jobs are under threat and that 2-6 million may be
lost over the next decade due to disruptive technologies such as
AI, robotics and blockchain. Concerns over potential job losses
have certainly filtered down to the WealthBriefing community.
But, as ever, the headlines belie a far more complex picture,
particularly as regards the wealth management sector.
Much is made of the fact that digital challenger banks have been able to launch with 90 per cent less headcount than traditional incumbents. Yet the fact remains that wealth management represents a very special niche, it being a highly complex, multi-disciplinary area of financial services and one that is predicated on high-touch personal service too.
As such, rather than fearing their replacement by AI, our expert
panellists argue that wealth management professionals should
instead look forward to their working lives being made very much
easier, and far more productive, through this technology.
Reservations even over robo-advice
Complexities exist even in areas where the “rise of the machines”
seems unassailable. Robo-advice undoubtedly represents a seismic
shift for the investment management industry, one that has
squeezed traditional providers’ profit margins and fuelled the
growth of passive investing to the point where many question the
ongoing existence of active management.
However, while 71 per cent of wealth managers may believe clients
are ready to take advice from robos, it seems doubtful that they
want to rely solely on them – despite what advocates may say
about likelihood of a passive, robo-managed portfolio performing
better – and at far less cost – than one actively managed by a
person.
It is telling that self-directed investing slid from 45 per cent
to 38 per cent between 2010 and 2016, a period where robos
stormed ahead in both number and sophistication. Here, it has
been suggested that the psychological difficulties of
“entrusting” large amounts of money to a technological interface
means that many are using these tools largely to assess their
investments before consulting a professional to actually manage
them. This seems very much borne out by research showing that
investors prize an understanding of their needs and goals, a
holistic overview and a willingness to explain analysis above all
else when seeking an advisor.
Relationship managers to remain central
The old adage that “people buy from people” seems to remain true,
and not just due to trust issues. Rather, it is that the human
element of wealth management is where much of its value
resides.
“The non-reduceable part of the human work in private banking is
really representing the client’s perspective, unpicking their
philosophy and attitudes to life and risk,” said Alessandro
Tonchia, Co-Founder of Finantix. “So, while more and more of
their ‘menial’ work will be eaten up by increasingly intelligent
systems, the relationship manager will still remain central as
the advocate of the client.”
As our experts observed, this advocacy is multi-faceted, with AI
holding out the potential for significant improvements in several
ways.
For Greg Davies, Head of Behavioural Science at Oxford Risk, one
of the most compelling use cases for AI is in helping advisors
synthesise the myriad information institutions hold on clients
with new details emerging in meetings to ensure that investment
suitability is properly addressed.
“Mentally processing all the existing and new client information
to arrive at the right recommendation for them can be incredibly
complicated with many moving parts,” he said. “AI can dynamically
process that information in real-time and do all the data
juggling to present the advisor with the most accurate, holistic
portrait the firm has of the client at that moment.”
Not only will AI save advisors the risk - and pain - of
performing all these calculations “live”, it can also flag up all
the important issues to be covered in the conversation. However,
Davies notes that the technology would still need to draw on a
human-designed conception of what the underlying suitability
framework is, just as really accurate risk-profiling requires the
“human touch” to interrogate and contextualise a client’s
professed attitudes and desires. As with so much in sophisticated
wealth management, risk-profiling is both an art and a
science.
Iron Man, not the Terminator
“The value of AI is less in the computer ‘giving the answer’ to
the client and more in it acting as an advisory support tool
helping the relationship manager make decisions,” Davies said.
“When it comes to advice, we should be thinking about AI in terms
of Iron Man, rather than the Terminator.”
Davies’ reference carries very useful notions of AI acting as a
kind of “cognitive prothesis” for advisors. The technology will
allow them to bring far more information about the client to bear
when making investment and financial planning recommendations; as
discussed later in this report, it will also be able to ensure
that these are compliant via machines learning (and automatically
keeping up to date with) all the regulatory constraints private
client practitioners have to work within.
The delivery of professional services of all kinds looks set to
be revolutionised by new technologies. But while the medical
applications of AI have garnered much attention, the greater
objectivity of this domain leads Tonchia to believe that it is
rather the legal professional which yields the most useful
parallels to wealth management.
“Legal and wealth are similarly about interpreting a client’s
often quite philosophical objectives, devising strategies to
achieve them, then articulating those into a tactical approach -
and both are incredibly rich in terms of the knowledge you need
to do that optimally,” he said. “An intelligent system can help a
lot in both sectors by validating courses of action, gathering
all the information and documents that are required and reducing
the risk of making mistakes.”
As the “fourth industrial revolution” gathers pace, Phil
Tattersall, Director in EY’s UK Wealth & Asset Management Data
and Analytics advisory practice, sees AI having a truly
transformative effect on wealth management as well as the wider
world. Yet this should be thought about in terms of a
multiplicity of gains, and not simply job losses.
An inflection point approaches
“If we plot human population on a graph over time, the inflection
point to geometric growth was enabled by the industrial
revolution, which massively increased productivity as we
augmented human and animal power with steam power,” he said. “The
promise of AI, and in particular Machine Learning (ML)
techniques, is to enable a similar inflection point in
productivity, this time via a massive augmentation in cognitive
power.”
Wealth managers are already investing heavily in AI technology.
EY’s Tattersall sees AI/ML techniques rapidly becoming embedded
in every single aspect of the wealth management value chain.
However, we can expect the application of AI to start in the
highest-value areas, namely, enhancing the investment process and
client experience and reducing business risk, all while
delivering the dramatic productivity gains the industry
needs.
According to our panel, the notion of moving up the value chain
is in fact one that all wealth management professionals should
keep in mind when considering the sector’s evolution and their
future role within it – particularly when we consider that,
hearteningly, 8 in 10 firms replacing roles with technology are
retraining or redeploying those being displaced.
If computers can perform a function optimally and without
intervention, then in time they undoubtedly will. But due to the
complex, multi-disciplinary and interpersonal nature of managing
wealth there seems to be relatively little - except in the most
workaday tasks - where humans seem likely to become entirely
redundant.
Instead, we can anticipate varying degrees of hybridisation
between man and machine, as the rest of this report describes.
This means that skilled relationship managers have little to
fear, and much to gain, from greater AI adoption across the
industry and that Chief Technology Officers should actually find
the technology an easier “sell” than many might have hitherto
feared – as long as the benefits for clients, firms and advisors
are made clear.
“There will be a need for humans to sell and persuade for many
decades to come,” concluded David Teten, Managing Partner of HOF
Capital. “People need to remember that the great majority of the
value of technology in general - and not just with AI - is in
augmenting professionals, not replacing them.”